Fengqi You
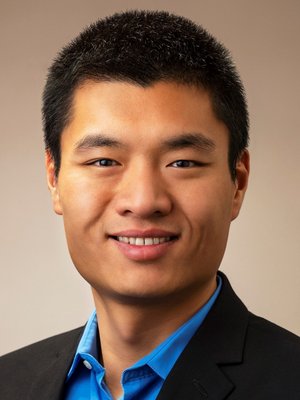
Prof. Dr. Fengqi You
Session: Systems Engineering and Computational Methods
Title: Quantum Computing and
Deep Learning for Process
Systems Optimization and
Data Analytics
Fengqi You is the Roxanne E. and Michael J. Zak Professor at Cornell University, and he is affiliated with the Graduate Fields of Chemical Engineering, Electrical and Computer Engineering, Operations Research and Information Engineering, Systems Engineering, Mechanical Engineering, Civil and Environmental Engineering, and Applied Mathematics. He also serves as Chair of Ph.D. Studies in Cornell Systems Engineering, Associate Director of Cornell Energy Systems Institute, and Associate Director of Cornell Institute for Digital Agriculture. He was on the faculty of Northwestern University and worked at Argonne National Laboratory before joining Cornell in 2016. He has an h-index of 67 and published over 200 refereed articles in journals, such as Science, Nature Sustainability, Nature Communications, and Science Advances. Some of his research results have been editorially highlighted in Science and Nature, featured on journal covers (e.g., Energy & Environmental Science), and covered by major media outlets (e.g., The New York Times, BBC, Reuters, The Wall Street Journal, Daily Mail, Newsweek, BusinessWeek, New Scientist, and National Geographic). His recent awards include American Institute of Chemical Engineers (AIChE) W. David Smith, Jr. Publication Award (2011), Northwestern-Argonne Early Career Investigator Award (2013), National Science Foundation CAREER Award (2016), AIChE Environmental Division Early Career Award (2017), AIChE Research Excellence in Sustainable Engineering Award (2017), Computing and Systems Technology (CAST) Outstanding Young Researcher Award from AIChE (2018), Cornell Engineering Research Excellence Award (2018), ACS Sustainable Chemistry & Engineering Lectureship Award (2018), AIChE Excellence in Process Development Research Award (2019), AIChE Young Investigator Award for Innovations in Green Process Engineering (2020), Mr. & Mrs. Richard F. Tucker Excellence in Teaching Award (2020), Curtis W. McGraw Research Award from American Society for Engineering Education (ASEE) (2020), and American Automatic Control Council (AACC) O. Hugo Schuck Award (2020), AIChE Sustainable Engineering Forum Education Award (2021), as well as a number of best paper awards. He is an editor of Computers & Chemical Engineering, an associate editor of AAAS journal Science Advances and IEEE Transactions on Control Systems Technology, a consulting editor of AIChE Journal, a guest editor of Energy and Renewable & Sustainable Energy Reviews, and an editorial board member of ACS Sustainable Chemistry & Engineering, Industrial & Engineering Chemistry Research, PRX Energy, etc. His research focuses on novel computational models, optimization algorithms, statistical machine learning methods, and multi-scale systems analytics tools for smart manufacturing, digital agriculture, energy systems, and sustainability. For more information about his research group: www.peese.org
Quantum Computing and Deep Learning for Process Systems Optimization and Data Analytics
The process systems engineering community has been developing computing and systems technologies (CASTs) and applying them to address chemical engineering problems across scales. Quantum computing and deep learning are emerging CASTs that have attracted growing interests from both academia and industry. This presentation will provide a brief introduction to the state-of-the-art of deep learning and quantum computing technologies, and discuss their potential applications in chemical engineering. Specifically, we will present the results of several collaborative research projects to illustrate the multi-scale applications of deep learning on molecular design, materials screening, sensor development, and energy systems control. In the second half of the presentation, we will introduce novel hybrid classical-quantum optimization algorithms that exploit the strengths of quantum computing techniques to address the computational challenges of important product-process systems engineering problems, ranging from molecular design, to manufacturing systems operations, and to supply chain optimization. The presentation will conclude with a novel deep learning model and quantum computing algorithm for efficient and effective fault diagnosis in chemical processes.